AI analytics enables companies to process complex data more efficiently and automate repetitive tasks, making scaling easy and frictionless. These tools can process thousands of API calls in minutes, providing critical information about brands, customer care, and competitive analysis in a matter of minutes. With AI-powered data analytics, businesses can make targeted decisions by processing vast amounts of data in real time, generating insights, and identifying patterns. This article explores how businesses can use AI for data analysis and the different AI tools available, such as AnswerRocket, ChatGPT, and Google Cloud AI.
Additionally, it discusses the limitations of AI and why it is unlikely to replace the need for human analysts entirely.
Data Analysis Using AI
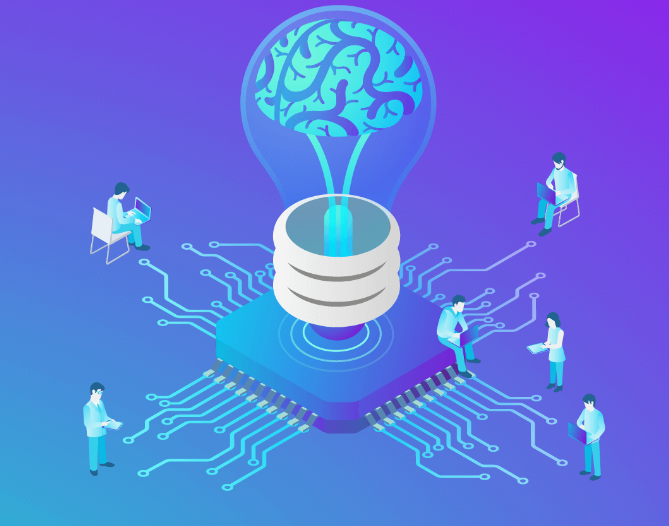
Applications In Various Industries
AI-driven data analysis has revolutionized numerous industries by providing advanced insights and predictive capabilities. By leveraging AI, businesses in healthcare, finance, and marketing have been able to enhance decision-making, improve operational efficiency, and gain a competitive edge.
Healthcare- Data Analysis Using AI
In the healthcare industry, AI-powered data analysis has played a pivotal role in disease diagnosis, treatment optimization, and patient care. Through machine learning algorithms, healthcare providers can analyze vast amounts of medical data to identify patterns, predict potential health issues, and personalize treatment plans for patients.
Finance- Data Analysis Using AI
AI has significantly impacted the finance sector by enabling sophisticated data analysis for risk assessment, fraud detection, and investment strategies. Financial institutions utilize AI algorithms to analyze market trends, assess creditworthiness, and automate decision-making processes, leading to more accurate predictions and reduced operational risks.
Marketing
AI-driven data analysis has transformed marketing strategies by enabling precise customer segmentation, personalized targeting, and real-time campaign optimization. Marketers harness AI technologies to analyze consumer behavior, predict purchasing patterns, and tailor marketing efforts to specific audience segments, resulting in improved customer engagement and higher conversion rates.
AI Techniques For Data Analysis
When it comes to AI data analysis online, various techniques enhance the process. These techniques leverage machine learning algorithms and natural language processing to extract valuable insights from vast datasets.
Machine Learning Algorithms
Machine learning algorithms play a crucial role in data analysis using AI. They enable computers to learn from data, identify patterns, and make decisions with minimal human intervention. Examples of machine learning algorithms include:
- Linear Regression
- Decision Trees
- Random Forest
- Support Vector Machines
- K-Means Clustering
Natural Language Processing
Natural Language Processing (NLP) is another vital aspect of AI-driven data analysis. NLP techniques allow machines to understand, interpret, and generate human language. Applications of NLP in data analysis include:
- Sentiment Analysis
- Text Summarization
- Named Entity Recognition
- Language Translation
- Speech Recognition
By leveraging these AI techniques for data analysis, organizations can gain deeper insights, improve decision-making, and drive business growth.
Challenges In Data Analysis With AI
When delving into data analysis with AI, several challenges emerge that need careful consideration. These challenges can impact the effectiveness and reliability of the analysis, ultimately influencing the decision-making process.
Data Privacy Concerns
Data privacy is a critical concern in the realm of data analysis with AI. The use of vast amounts of data, including sensitive personal information, raises questions about how this data is collected, stored, and utilized. Ensuring compliance with data protection regulations and maintaining the confidentiality of data are paramount in safeguarding individuals’ privacy.
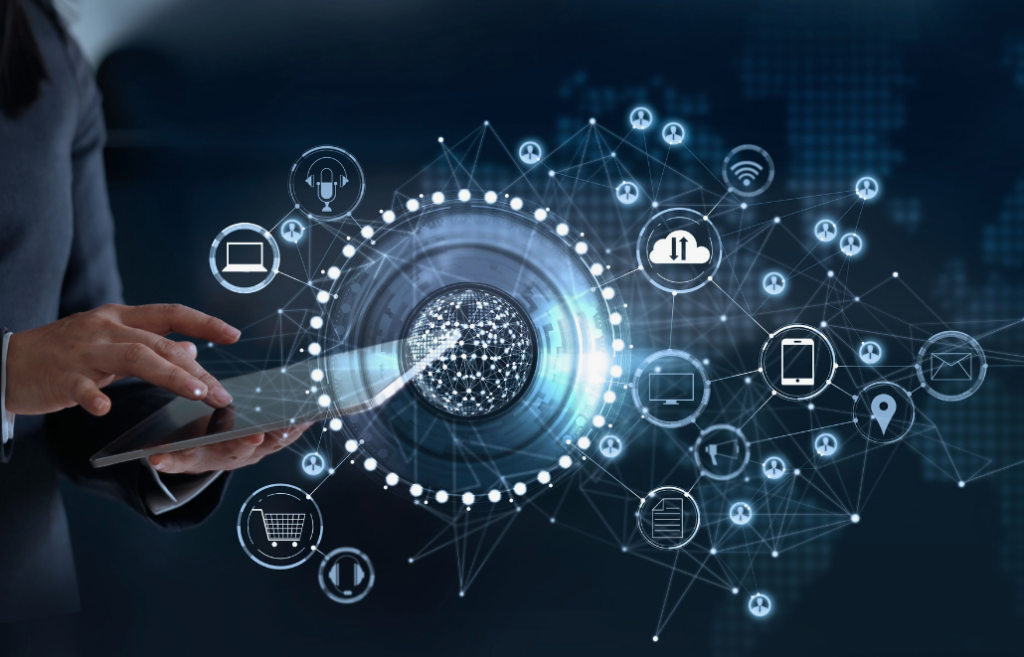
Interpretability of AI Models
The interpretability of AI models presents another significant challenge in data analysis. Understanding how AI algorithms arrive at their conclusions is crucial for building trust in the analysis results. Lack of transparency in AI decision-making processes can hinder the acceptance of insights derived from these models.
Best Practices
When it comes to data analysis using AI, it’s essential to follow best practices to ensure accurate and valuable insights. From data preprocessing to model evaluation, implementing the right strategies can significantly impact the quality of your analysis. Let’s delve into the best practices for leveraging AI tools for data analysis and automating data analysis using artificial intelligence.
Data Preprocessing
Data preprocessing is a crucial step in preparing the data for analysis. It involves cleaning, transforming, and organizing the data to ensure its suitability for AI-based analysis. Here are some best practices for data preprocessing:
- Data Cleaning: Remove outliers, handle missing data, and eliminate inconsistencies to ensure the accuracy of the dataset.
- Feature Scaling: Normalize or standardize the features to bring them to a similar scale, preventing certain features from dominating the analysis.
- Dimensionality Reduction: Use techniques like PCA (Principal Component Analysis) to reduce the number of features while retaining essential information.
Model Evaluation
Model evaluation is essential to assess the performance and effectiveness of the AI models used for data analysis. Employing the right evaluation techniques is critical for deriving reliable insights. Here are some best practices for model evaluation:
- Cross-Validation: Implement cross-validation techniques to validate the model’s performance across different subsets of the data, reducing overfitting.
- Performance Metrics: Utilize appropriate metrics such as accuracy, precision, recall, and F1 score to evaluate the model’s predictive capabilities.
- Ensemble Methods: Consider ensemble methods like bagging and boosting to improve the overall predictive performance of the models.
Future Trends
The future trends in data analysis using AI are shaping the way businesses make decisions and gain insights from their data. As technology continues to evolve, several key areas are emerging as game-changers, including Automated Machine Learning and Explainable AI. These developments are revolutionizing the way organizations harness the power of data to drive innovation and achieve competitive advantages.
Automated Machine Learning
Automated Machine Learning (AutoML) is a significant trend that is transforming the landscape of data analysis. It involves the use of AI and machine learning algorithms to automate the process of model selection, feature engineering, and hyperparameter tuning. This enables organizations to expedite the development of machine learning models, even with limited expertise, thereby enhancing productivity and accelerating time-to-insight.
Explainable AI
Explainable AI is another crucial trend in data analysis, particularly in the context of AI model interpretability and transparency. It focuses on developing AI systems that can provide clear explanations for their decisions and predictions, thereby enhancing trust and understanding among users. With the increasing adoption of AI in critical decision-making processes, explainable AI is becoming indispensable for ensuring accountability and ethical use of AI.
Ethical Considerations
When automating data analysis using artificial intelligence (AI), it’s crucial to consider the ethical implications that come with leveraging advanced technology to make data-driven decisions. Ethical considerations play a pivotal role in ensuring that AI-driven data analysis is fair, transparent, and free from biases. In this context, it’s essential to address issues such as bias in AI, fairness, and transparency.
Bias In AI
One of the primary ethical considerations in AI-driven data analysis is the potential for bias. AI algorithms can inadvertently perpetuate biases present in the training data, leading to discriminatory outcomes. This can have significant implications for decision-making processes, especially in areas such as hiring, lending, and criminal justice. Addressing and mitigating bias in AI is essential for fostering equitable and unbiased data analysis.
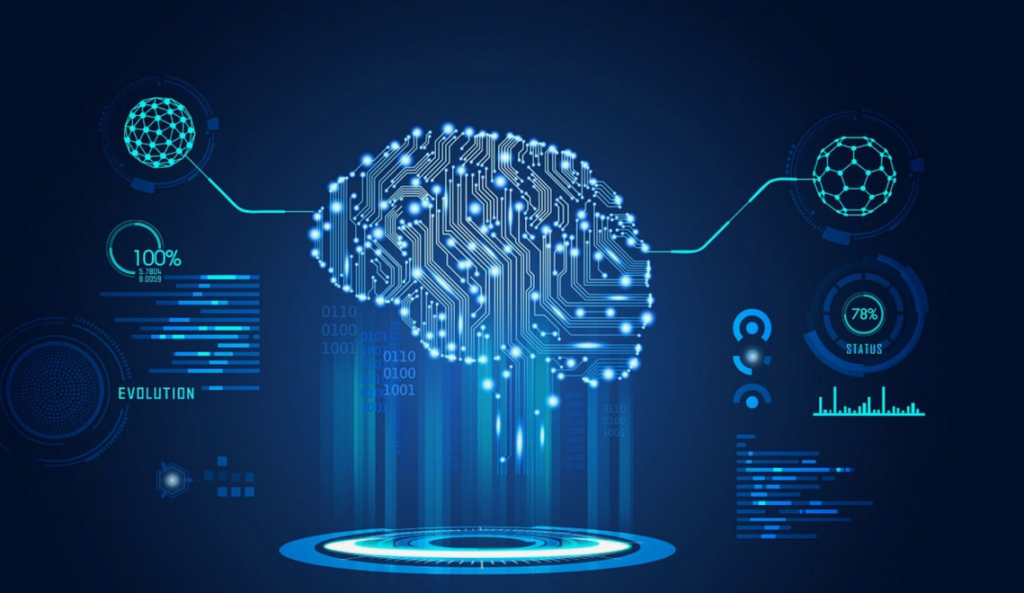
Fairness and Transparency
Ensuring fairness and transparency in AI data analysis is paramount for building trust and accountability. Transparency in the AI models and algorithms used for data analysis is vital for stakeholders to understand the decision-making process. Fairness encompasses the equitable treatment of individuals and groups, safeguarding against discriminatory outcomes. By prioritizing fairness and transparency, organizations can uphold ethical standards in AI-driven data analysis.
Conclusion
Enhance your data analysis with AI tools for streamlined processes and valuable insights. AI analytics is revolutionizing decision-making with automation and efficiency. Dive into the world of AI-powered data analysis to stay ahead in the competitive landscape. Explore the endless possibilities AI offers for your business growth and success.